Building an AIs that are able to communicate with human beings, with understanding “emotions evoked by humor” and “a sense of justice according to moral standards”, is one of the ultimate goals of researchers. Conversely, automatically generate texts and contents that make humans feel above mentioned emotions would be potentially demanding.
Humor enriches human life, but some jokes fail to entertain people due to their lack of morality. In the modern society where “humor” that treats one’s appearance as a pig attracts the attention of society, there is no end to the number of serious incidents and accidents that result in suicide and depression due to morally offensive postings against the backdrop of the penetration of social networking services. It can be said that there is a latent demand for humor that is both moral and funny.
In this paper, we propose a mechanism that can select humor based on moral criteria for “moral joke generation”. First, we generate candidate jokes. Specifically, we use an N-gram corpus constructed from a vast amount of text on the Web, and generate candidate jokes using various template patterns based on statistics that can be used as jokes. Here, we also use template patterns that take into account Theory of Mind (ToM), in which we infer what others are thinking and feeling, and based on that inference, analogize what they would do in a particular situation. The next step is to select the jokes that are generated. Specifically, we train Long-Short Term Memory (LSTM), a recurrent neural network, on the Moral Foundations Twitter Corpus, which consists of 35,000 tweets with moral values. This is used for joke selection.
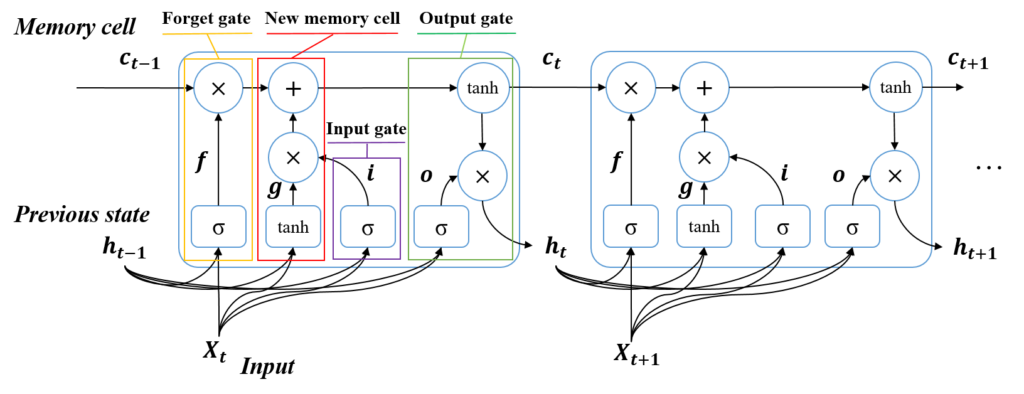
Experimental results obtained from Best-Worst Scaling show that this method can generate jokes with moral category labels. Jokes about classifiers classified as Loyalty and Authority, which are considered “morally good” in our study, are superior to jokes about Fairness, Purity, Harm, Cheating, and Degradation. Jokes about the classifiers Fairness, Purity, Harm, Cheating, and Degradation were found to be predominantly funny.