Decoding human mental content (e.g., selecting “cat” from multiple categories when a subject is actually seeing a cat on a screen) solely from fMRI brain activity is an important objective for the neuroscience and brain-machine interface fields. However, because individual brains differ significantly, it is extremely difficult to interpret the mental content of one subject based on data from another subject.
Domain adaptation (DA) is a transfer learning method in which a model trained on a source distribution is applied in the context of a different target distribution. In this study, we first constructed a deep learning model that can decode the mental contents of an individual and then examined the applicability of DA.
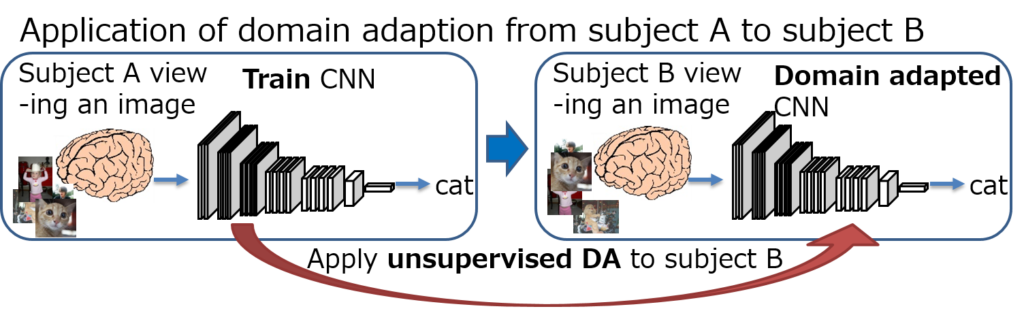
We first constructed a deep learning model to decode the mental contents of individuals. We then examined the applicability of DA by comparing the developed model to other methods without DA, namely a linear SVM and CNN. With accuracy exceeding the chance rate, we observed that most DA subjects significantly outperformed non-DA subjects.
We presented the above work under the title of “Unsupervised domain adaptation for category prediction in fMRI brain decoding”
Hiroaki Yamane, Atsushi Kanehira, Tatsuya Harada
Computer Assisted Radiology and Surgery (CARS) June 2020